
Demand response (DR) strategies focus on flexibilizing energy demand. This can be achieved through simulation-based solutions that also promote solution validation.Ī continuing increase in energy supply from variable renewable energy (VRE) sources requires new strategies to match energy supply and demand. Through holistic solutions, it is possible to access to which extent introducing energy flexibility measures may propagate through the production system. Hence, there is a need to focus on solutions that promote a holistic view of the production flow. Based on the scoping review analysis and results, it was found that there is a gap between the evaluation of energy flexibility and the impact on the production flow. Furthermore, the result indicates that optimization is the main evaluation method. Meanwhile, the results reveal a tendency to focus on evaluating the potentials in a single process within the industrial facility. The result indicates that the field of evaluating the potential of industrial energy flexibility is matured with an increasing research interest. Through a systematic quantitative and qualitative review, 26 essential publications fulfill the criteria. The scoping review was conducted in the last quarter of 2019, and 855 publications were found through related databases.
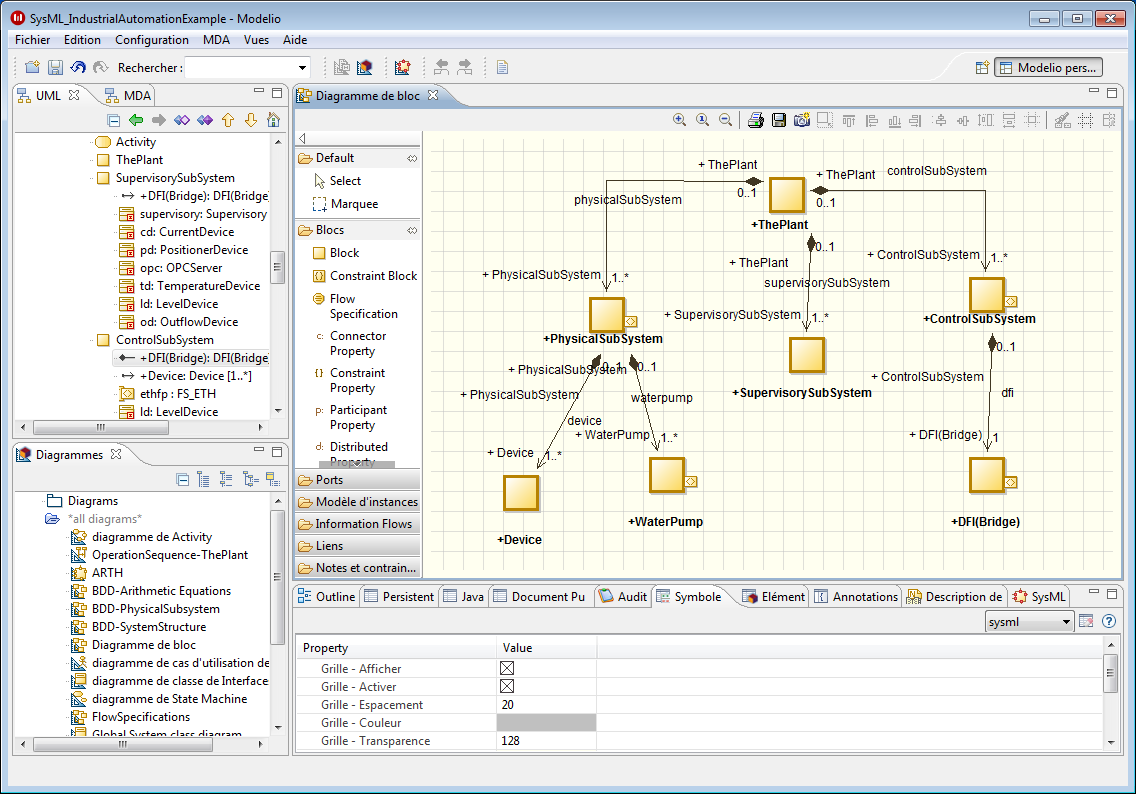
This paper applies a scoping review methodology and presents the findings for uncovering industrial energy flexibility. It is vital to conduct the evaluation of industrial energy flexibility potential to provide knowledge to relevant market players about the energy potential with economic gain through unlocking flexibility. This paper was with the authors 2 weeks for 1 revision.). (Received in February 2021, accepted in April 2021. The proposed method was proved effective through experiments. After that, the authors proposed a dynamic job-shop production control method based on deep reinforcement learning, and explained the collaboration strategy for multiple subsystems. Then, deep reinforcement learning was introduced to job-shop production control system to transform the dynamic job-shop production control problem. Firstly, a multi-objective optimization model was established for the production control system of dynamic job-shop. Therefore, this paper probes into the dynamic job-shop production control problem based on deep reinforcement learning and rule scheduling.
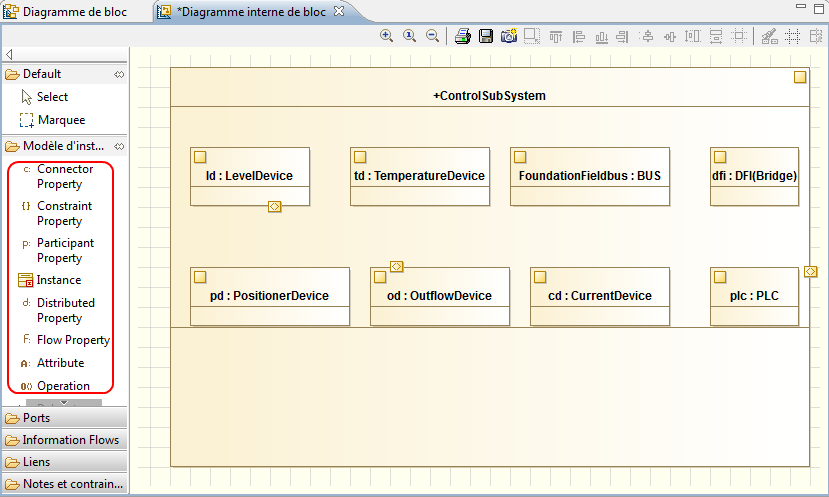
The desired control objectives are not easily achieved for job-shop production control problems with dynamic changes. However, the existing studies have not provided an evaluation mechanism to reasonably measure the control efficiencies of different plans. As intelligent and precision manufacturing becomes the trend of industrial production, it is of practical significance to study the job-shop production control.
